In 2024, open-source Generative AI (GenAI) is not just growing—it's exploding. Meta's Llama model family has seen downloads skyrocket to 350 million, a massive tenfold increase from the previous year. In July 2024 alone, Llama models were downloaded over 20 million times, cementing its position as the leading open-source model family (Meta AI, 2024).
These numbers signal a seismic shift in enterprise AI strategy. In fact, a multi-month study by Andreessen Horowitz uncovered “16 Changes to the Way Enterprises Are Building and Buying Generative AI1,” concluding enterprises are planning to increase their GenAI spend by two to five times in 2024, with a focus on moving more workloads into production, adopting multiple models, and increasingly shifting towards open source — with many AI leaders targeting a 50/50 split, up from the 80% closed/20% open split in 2023.
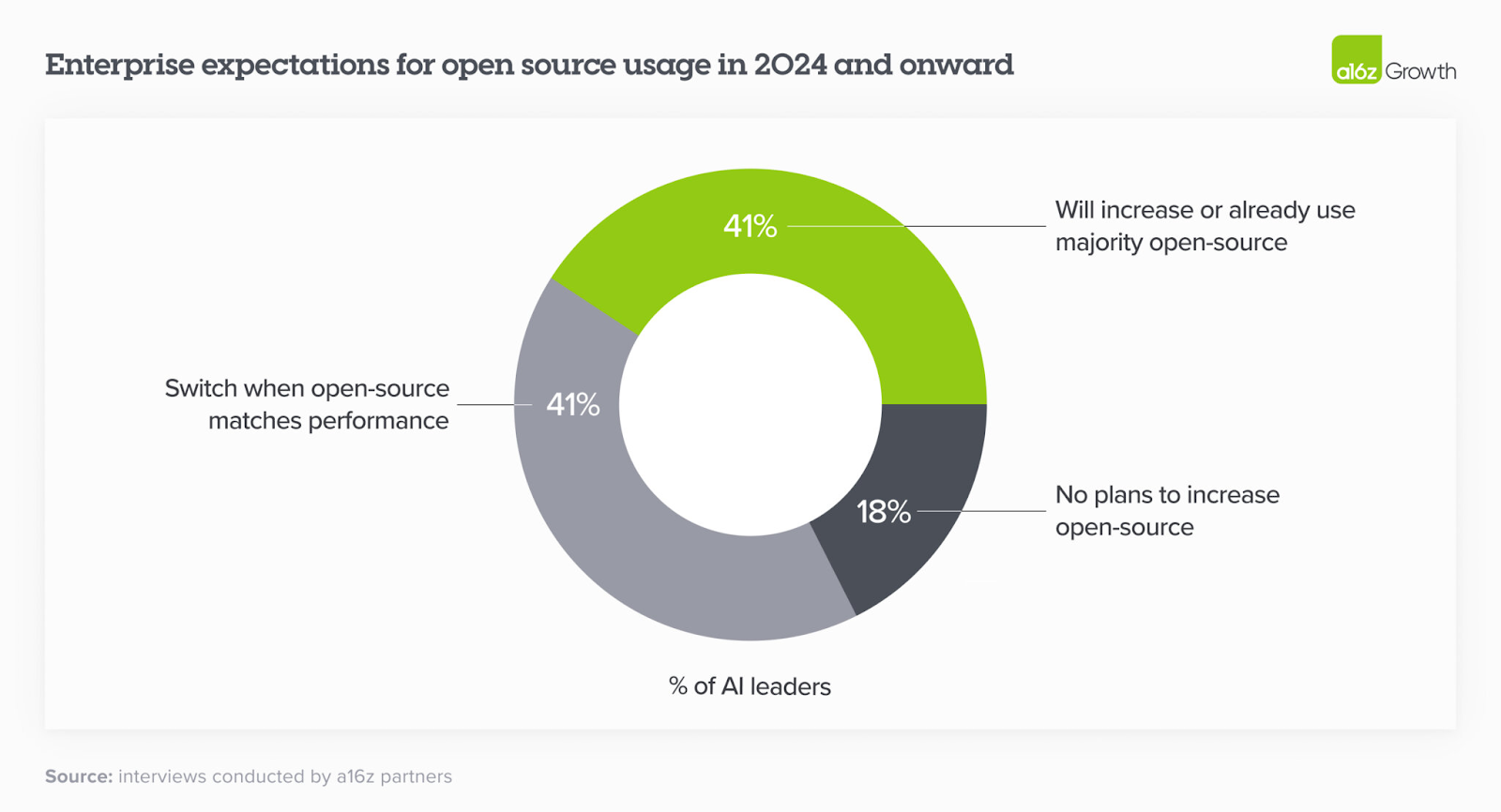
‍
The Open Source Advantage
Why are enterprises flocking to open-source GenAI?Â
The answer lies in a powerful combination of flexibility, control, and innovation potential. These models are becoming the backbone of diverse applications across the enterprise, from enhancing customer care to streamlining document processing, and from fueling creative endeavors to supercharging internal knowledge management.
Open-source models offer several key benefits for enterprises:
- Avoiding vendor lock-in: Companies can adapt and modify the models to suit their specific needs without being tied to a single provider.
- Greater control over data: Enterprises can implement these models within their own infrastructure, ensuring better oversight of sensitive information.
- Faster scaling: The ability to customize and optimize open-source models often allows for more efficient scaling across diverse use cases.
However, harnessing the full potential of open-source GenAI requires more than just access to the models themselves. Enterprises need robust infrastructure and management tools to effectively deploy and scale these models. This is where platforms like Run:ai come into play. By providing seamless integration with popular model hubs such as Hugging Face, Run:ai empowers enterprises to accelerate their GenAI deployment while maintaining the control and flexibility that make open-source models so attractive. This approach allows organizations to bridge the gap between cutting-edge AI capabilities and enterprise-grade infrastructure requirements.
The New Enterprise Reality
This shift isn't just about adopting new technology; it's about redefining the very fabric of enterprise operations.Â
The rapid adoption of models like Llama, Mistral, and Stable Diffusion reflects a broader trend: enterprises need solutions that are adaptable and customizable to their specific needs. Open-source models are leading this charge by enabling organizations to rapidly develop AI solutions that fit within their operational frameworks, while still retaining control over security, data, and infrastructure.
The key advantage of open-source models is the flexibility they provide. Whether for creative applications or robust NLP tasks, enterprises can customize and deploy open source models quickly across different infrastructure environments. This is crucial for enterprises that need to balance innovation with security and compliance, especially when scaling GenAI across departments or regions.
From Cold Starts to Full Scale: The Technical Challenge
As enterprises scale their GenAI initiatives, they face significant challenges that require careful planning and optimization. To address these challenges and provide actionable insights, we're launching a blog series that will explore these key areas, among other topics:
- Open Source GenAI, Your Infrastructure, Your Way: We'll examine how enterprises can leverage cutting-edge open-source models while maintaining control over their infrastructure to support fast experimentation without compromising security or flexibility.
- Containing the Cold Start Challenge: We'll dive into strategies for reducing cold start delays that can slow down mission-critical applications, focusing on resource management and model caching to minimize latency for real-time inference.
- Scaling the Entire AI Lifecycle: We'll provide guidance on how to scale every phase of the AI lifecycle—from development to production. Topics include intelligent resource allocation, managing workloads across training, development, and inference, and maximizing efficiency in hybrid environments.
This series will offer actionable insights to help enterprises address these technical challenges and fully capitalize on the benefits of GenAI. By tackling these critical areas, companies can gain a competitive edge in their AI implementations and unlock the full potential of open-source GenAI.
Looking Ahead: Assessing the Choices and Trade-offs Across the Model Landscape
As we look to the future, the enterprise GenAI landscape is rich with options, both proprietary and open-source. Understanding the advantages and trade-offs is critical for making informed decisions about which models to adopt and how to integrate them into existing workflows and business operations.
The next blog in this series will provide an in-depth overview of the GenAI model landscape, discussing both proprietary and open-source options like Llama, Mistral, and Stability AI. We'll explore the infrastructure trade-offs that enterprises need to consider when selecting a model, including:
- Performance vs. Flexibility: How customizable and adaptable the model is versus its computational demands.
- Scalability: The ease of scaling across hybrid environments.
- Security & Compliance: Balancing innovation with the need for data security and regulatory compliance.
By staying informed about these developments and carefully considering their options, enterprises can position themselves to fully capitalize on the benefits of open-source GenAI, driving innovation and maintaining a competitive edge in an increasingly AI-driven business landscape.
—
This blog post is the first in a series exploring the integration of open-source Generative AI in enterprise environments. Follow along as we delve into the technical challenges and strategic opportunities that define this new phase of AI adoption.
‍
1 Wang, S., & Xu, S. (n.d.). 16 Changes to the Way Enterprises Are Building and Buying Generative AI. Andreessen Horowitz. Retrieved September 20, 2024, from https://a16z.com/generative-ai-enterprise-2024/‍